论文题目:RAMP: Retrieval-Augmented MOS Prediction via Confidence-based Dynamic Weighting
作者:Hui Wang (Nankai University); Shiwan Zhao (Independent Researcher); Xiguang Zheng (Kuaishou Technology); Yong Qin (Nankai University)
通讯作者:Yong Qin (Nankai University)
录用会议:Interspeech 2023
论文概述:Automatic Mean Opinion Score (MOS) prediction is crucial to evaluate the perceptual quality of the synthetic speech. While
recent approaches using pre-trained self-supervised learning (SSL) models have shown promising results, they only partly address the data scarcity issue for the feature extractor. This leaves the data scarcity issue for the decoder unresolved and leading to suboptimal performance. To address this challenge, we propose a retrieval-augmented MOS prediction method, dubbed RAMP, to enhance the decoder’s ability against the data scarcity issue. A fusing network is also proposed to dynamically adjust the retrieval scope for each instance and the fusion weights based on the predictive confidence. Experimental results show that our proposed method outperforms the existing methods in multiple scenarios.
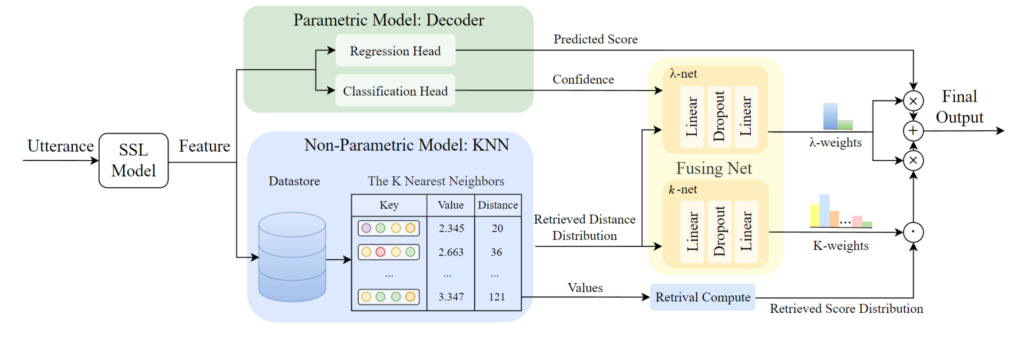
论文题目:Supervised Contrastive Learning with Nearest Neighbor Search for Speech Emotion Recognition
作者:Xuechen Wang, Shiwan Zhao, Yong Qin
通讯作者:Yong Qin
录用会议:Interspeech 2023
论文概述:Speech Emotion Recognition (SER) is a challenging task due to limited data and blurred boundaries of certain emotions. In
this paper, we present a comprehensive approach to improve the SER performance throughout the model lifecycle, including pre-training, fine-tuning, and inference stages. To address the data scarcity issue, we utilize a pre-trained model, wav2vec2.0. During fine-tuning, we propose a novel loss function that combines cross-entropy loss with supervised contrastive learning loss to improve the model’s discriminative ability. This approach increases the inter-class distances and decreases the intra-class distances, mitigating the issue of blurred boundaries. Finally, to leverage the improved distances, we propose an interpolation method at the inference stage that combines the model prediction with the output from a k-nearest neighbors model. Our experiments on IEMOCAP demonstrate that our proposed methods outperform current state-of-the-art results.
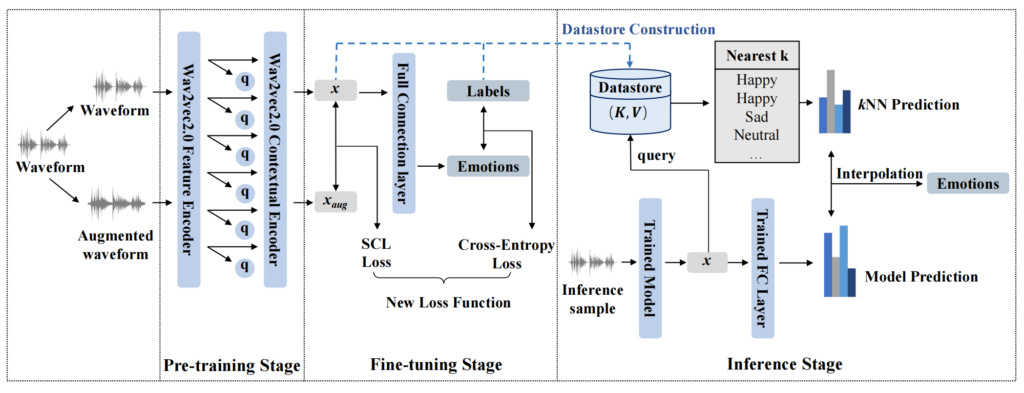