论文题目:KNN-CTC: Enhancing ASR via Retrieval of CTC Pseudo Labels
作者:Jiaming Zhou, Shiwan Zhao, Yaqi Liu, Wenjia Zeng, Yong Chen, Yong Qin
通讯作者:Yong Qin
录用会议:ICASSP 2024
论文链接:https://ieeexplore.ieee.org/abstract/document/10447075
论文概述:The success of retrieval-augmented language models in various natural language processing (NLP) tasks has been constrained in automatic speech recognition (ASR) applications due to challenges in constructing fine-grained audio-text datastores. This paper presents kNN-CTC, a novel approach that overcomes these challenges by leveraging Connectionist Temporal Classification (CTC) pseudo labels to establish frame-level audio-text key-value pairs, circumventing the need for precise ground truth alignments. We further introduce a “skip-blank” strategy, which strategically ignores CTC blank frames, to reduce datastore size. By incorporating a k-nearest neighbors retrieval mechanism into pretrained CTC ASR systems and leveraging a fine-grained, pruned datastore, kNN-CTC consistently achieves substantial improvements in performance under various experimental settings. Our code is available at https://github.com/NKU-HLT/KNN-CTC.
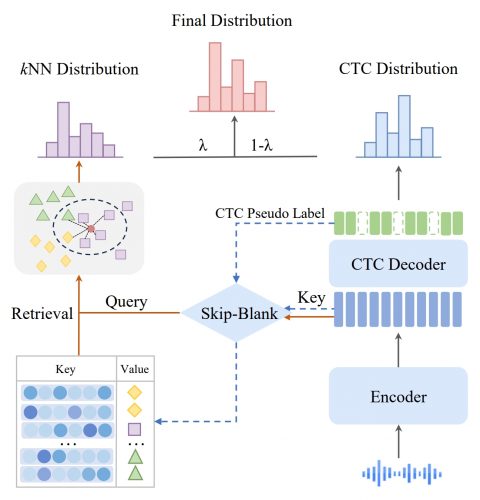
论文题目:Fine-grained disentangled representation learning for multimodal emotion recognition
作者:Haoqin Sun, Shiwan Zhao, Xuechen Wang, Wenjia Zeng, Yong Chen, Yong Qin
通讯作者:Yong Qin
录用会议:ICASSP 2024
论文链接:https://ieeexplore.ieee.org/abstract/document/10447667
论文概述:Multimodal emotion recognition (MMER) is an active research field that aims to accurately recognize human emotions by fusing multiple perceptual modalities. However, inherent heterogeneity across modalities introduces distribution gaps and information redundancy, posing significant challenges for MMER. In this paper, we propose a novel fine-grained disentangled representation learning (FDRL) framework to address these challenges. Specifically, we design modality-shared and modality-private encoders to project each modality into modality-shared and modality-private subspaces, respectively. In the shared subspace, we introduce a fine-grained alignment component to learn modality-shared representations, thus capturing modal consistency. Subsequently, we tailor a fine-grained disparity component to constrain the private subspaces, thereby learning modality-private representations and enhancing their diversity. Lastly, we introduce a fine-grained predictor component to ensure that the labels of the output representations from the encoders remain unchanged. Experimental results on the IEMOCAP dataset show that FDRL outperforms the state-of-the-art methods, achieving 78.34% and 79.44% on WAR and UAR, respectively.
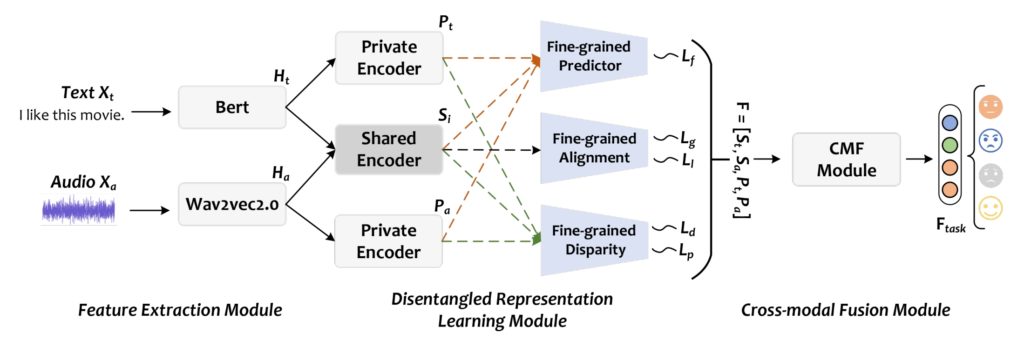